Simulation of extremely low flows in the Rhine River
The hydrological model WRF-Hydro was developed to improve the representation of land-atmosphere interactions with the meteorological model Weather Research Forecast (WRF). Over the past decade, researchers have extensively tested WRF-Hydro in various river basins for different purposes, particularly to investigate flood events. Lee et al. (2022) have successfully applied the model to simulate low water in South Korea, with the model results being in good agreement with the observed discharges. In addition, output variables can be used to identify drought periods based on drought indices. This conclusion confirms that the WRF-Hydro model is suitable for evaluating river navigability under extreme drought conditions.
As part of the CEDIM project Impact of recent and future drought events on river discharge and fluvial transport sector for the Rhine River, the simulated discharge during prolonged dry periods on the Rhine was assessed using WRF-Hydro. Meteorological input was obtained from the European Centre for Medium-Range Weather Forecasts (ECMWF) Reanalysis Version 5 (ERA5). The model was calibrated by comparing runoff simulations with water level observations from the Global Runoff Data Centre (GRDC).
To calibrate the simulated discharge values, three parameters recommended in the literature were selected. The first parameter is the surface retention depth (RETDEPRTFAC), which determines the water movement in the channel from upstream to downstream. This condition only occurs when the water impounded in the channel exceeds the depth of the parameter (Gochis, 2020). The second parameter, the retention factor (refkdt), influences surface infiltration and determines the amount of precipitation that enters the soil (Yucel, 2016). The infiltration parameter (slope) scales the amount of water that infiltrates into the lowest soil layer, as stated by Rummler (2018). It was suggested that the parameters should not be kept constant over the entire area, but that a spatial distribution of the individual parameters should be achieved using land use information (Rummler, 2018). This approach can significantly accelerate the calibration process and thus improve the results of the discharge values in a complex catchment area. Figure 1 shows the distribution of the three spatially distributed parameters used in the entire catchment.
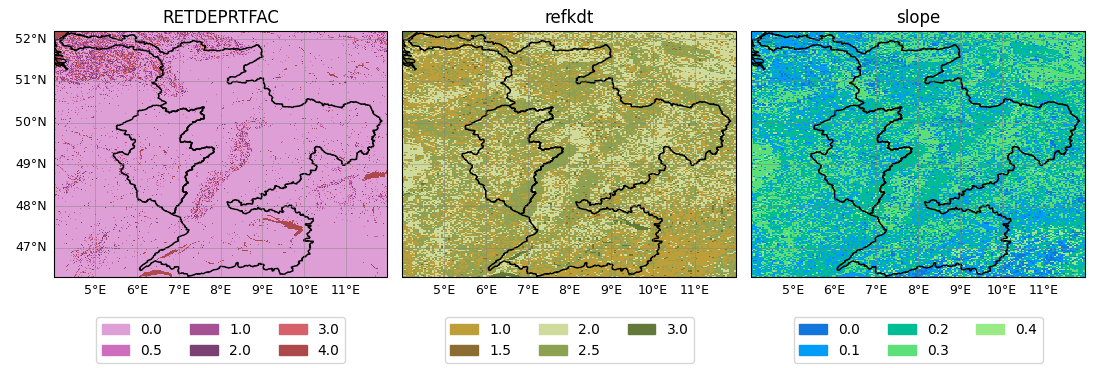
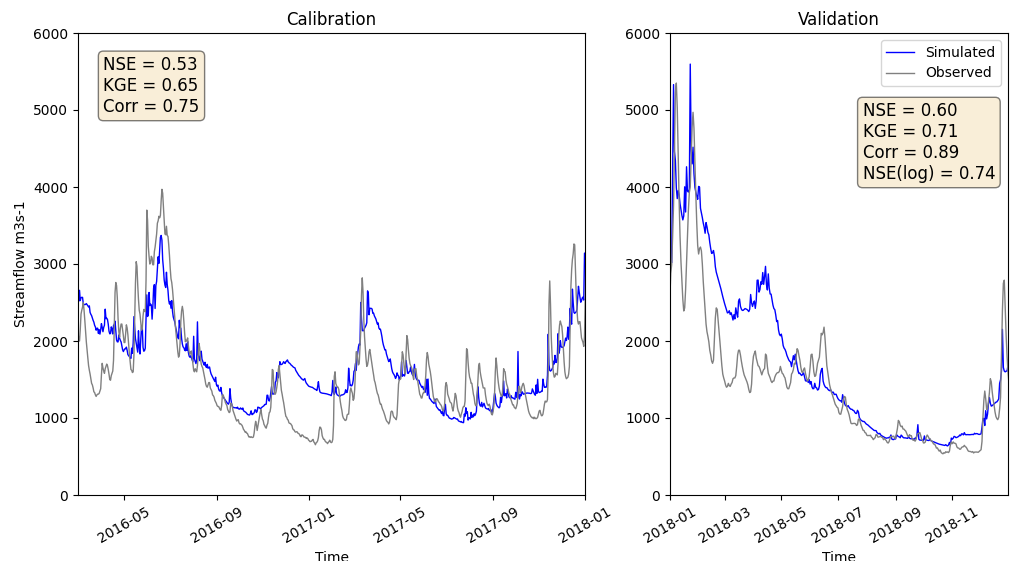
Figure 2 illustrates that the hydrograph of the calibrated model aligns well with the observed data. The statistical comparison values between the model and the observations at the Kaub station fall within an acceptable range of values. Knoben et al. (2019) recommend evaluating the simulated results using the Nash-Sutcliffe efficiency (NSE) and Kling-Gupta efficiency (KGE). The authors state that the values can be considered acceptable if NSE > 0.5 or KGE > 0.3. This is true for the mean values of both periods. Additionally, the results demonstrate an acceptable logarithmic Nash-Sutcliffe efficiency (NSE(log)) value. This value emphasizes the importance of the minimum flow and demonstrating the model's capability to accurately capture low water levels.
References
- Gochis, D. M. (2020). WRF-Hydro V5.1.1 Documentation. Boulder, USA: National Center for Atmospheric Research. Retrieved from National Center for Atmospheric Research. Research Application Laboratory: https://ral.ucar.edu/sites/default/files/public/WRFHydroV511TechnicalDescription.pdf
- Knoben, W. J. M., Freer, J. E., and Woods, R. A. (2019). Technical note: Inherent benchmark or not? Comparing Nash–Sutcliffe and Kling–Gupta efficiency scores, Hydrol. Earth Syst. Sci., 23, 4323–4331, https://doi.org/10.5194/hess-23-4323-2019
- Lee J., K. Y. (2022). Assessing the characteristics of recent drought events in South Korea using WRF-Hydro. Journal of Hydrology (607), 127459. doi:https://doi.org/10.1016/j.jhydrol.2022.127459
- Rummler T., Arnault J., Gochis D., & Kunstmann H. (2019). Role of lateral terrestial water Flow on the regional water cycle in a complex terrain region: investigation with a fully coupled model. Journal of Geophysical Research: Atmospheres, 124. 507-529. https://doi.org/10.1029/2018JD029004
- Yucel I., O. A. (2015). Calibration and evaluation of a flood forecasting system: Utility of numerical weather prediction model, data assimilation and satellite-based rainfall. Journal of Hydrology. Volume 523, 49-66. doi:https://doi.org/10.1016/j.jhydrol.2015.01.042
Associated institute at KIT: Institute of Meteorology and Climate Research Troposphere Research (IMKTRO)
Author: Andrea Campoverde Márquez (March 2023)